News
- Jan 2021: Code and dataset released
- Aug 2020: Catch our presentation at the Perception for Autonomous Driving workshop at ECCV 2020
- June 2020: AutoLay accepted to IROS 2020
- March 2020: Check out MonoLayout, where this all started
Dataset
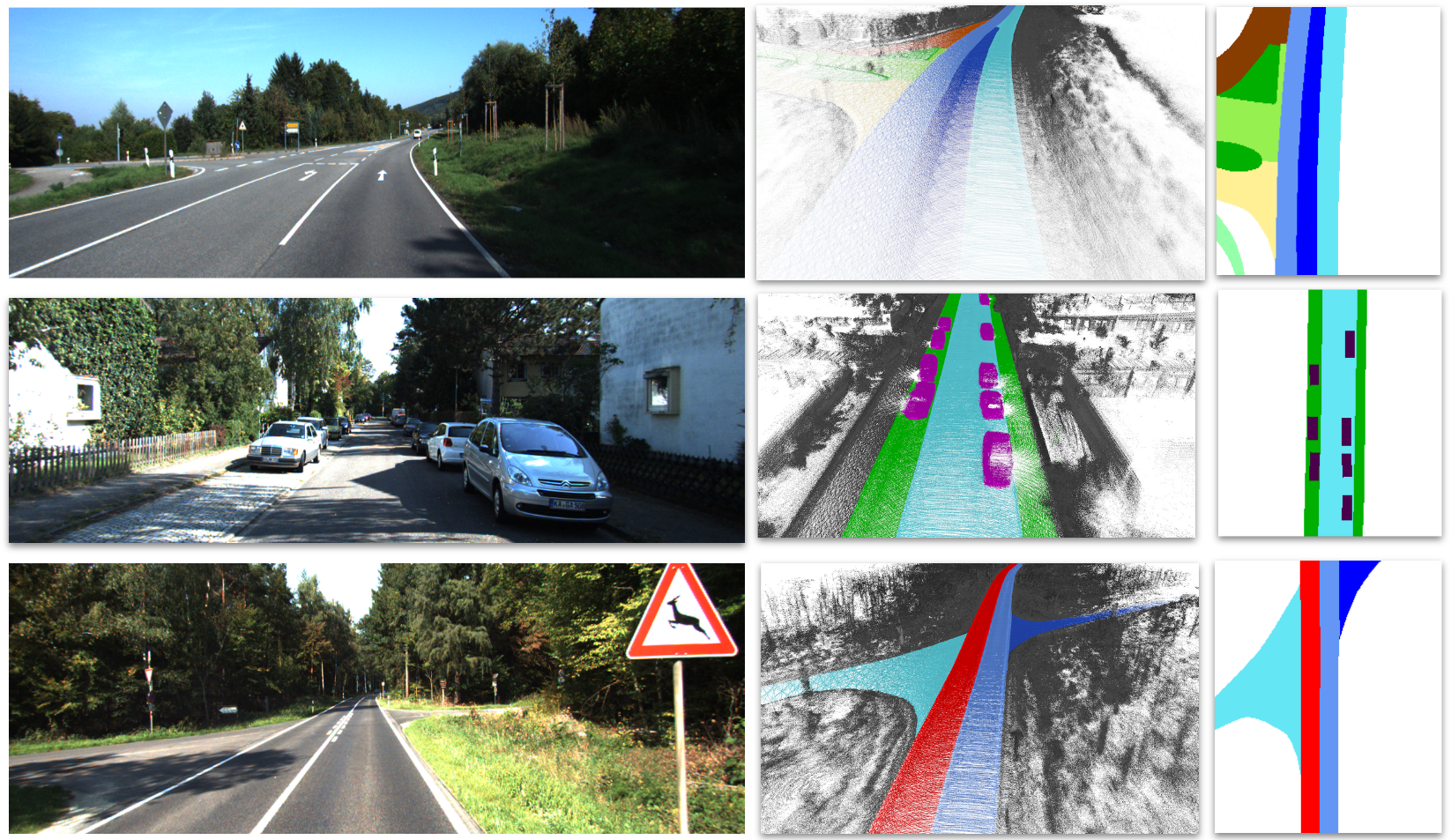
Sequences
Distance (Km)
Frames
Tasks
Given an image, or a sequence of images, we define the task of amodal layout estimation as estimating a bird's-eye view layout of the scene. This layout is amodal in that we also reason about the occupancies and semantics of regions of the scene that are outside the field of view or are occluded in the image.
- Road Layout
- Vehicle Layout
- Lanes Layout
- Ego-lane Layout
- Other lanes Layout
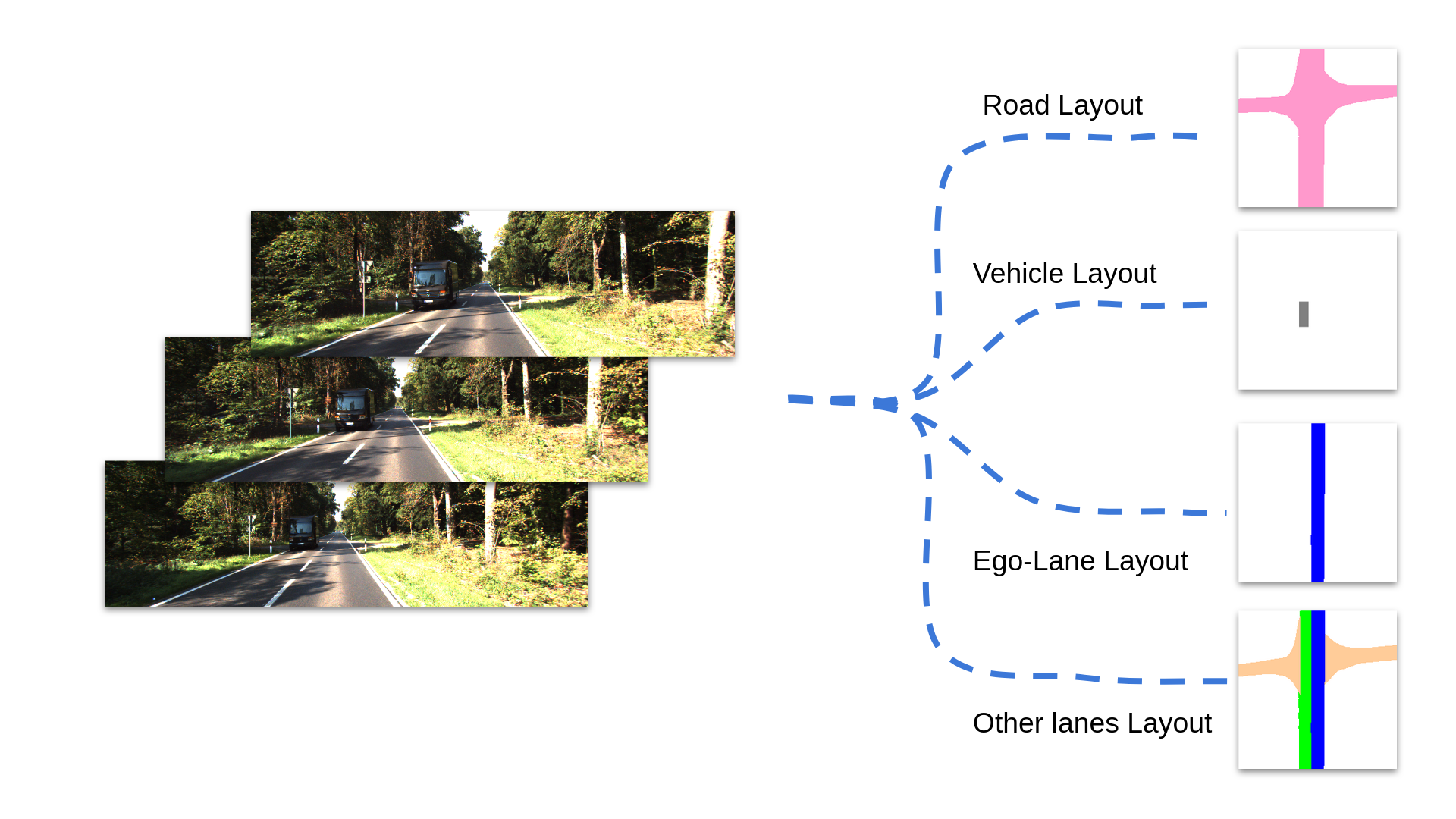
Download
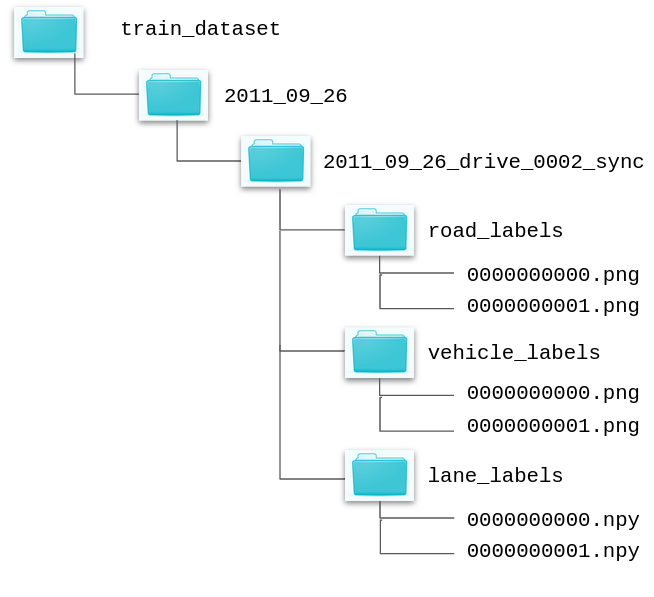
The Autolay dataset contains road, vehicle and lane layouts with the hierarchy same as the KITTI-RAW dataset. Our train split contains layout data for 24 sequences belonging to the City, Residential, and Road categories. The test split contains layouts datafor 22 sequences from the same categories.
The corresponding RGB image for any layout can be found in the image_02
folder of the particular sequence in the RAW dataset. The road and the vehicle layouts are available as .png
files, and the lane layouts are available as .npy
files containing semantic labels for each lane. More information regarding the semantic labels can be found in this readme file.
Download the Autolay train split
Download the Autolay test split
Papers
Check out our paper for more dataset statistics, results and baselines.
If you find this work useful, please use the following BibTeX entry for citing us!
@inproceedings{mani2020autolay,
title={AutoLay: Benchmarking amodal layout estimation for autonomous driving},
author={Mani, Kaustubh and Shankar, N Sai and Jatavallabhula, {Krishna Murthy} and Krishna, {K Madhava}},
booktitle={IEEE International Conference on Intelligent Robotics and Systems (IROS)},
year={2020},
}

You might also find MonoLayout---our earlier work on amodal scene layout estimation---useful and/or interesting.
@inproceedings{mani2020monolayout,
title={MonoLayout: Amodal scene layout from a single image},
author={Mani, Kaustubh and Daga, Swapnil and Garg, Shubhika and Narasimhan, Sai Shankar and Krishna, Madhava and Jatavallabhula, {Krishna Murthy}},
booktitle={The IEEE Winter Conference on Applications of Computer Vision},
year={2020},
}
If you use our work, please also cite the KITTI Vision Benchmark Suite
@article{Geiger2013IJRR,
author = {Andreas Geiger and Philip Lenz and Christoph Stiller and Raquel Urtasun},
title = {Vision meets Robotics: The KITTI Dataset},
journal = {International Journal of Robotics Research (IJRR)},
year = {2013},
}
Team
Graduate Student, IIIT Hyderabad
Research Assistant, IIIT Hyderabad
PhD candidate, Mila
Professor, IIIT Hyderabad